In mid-March, a one-minute video of Ukraine’s president Volodymyr Zelensky emerged first on social media and subsequently on a Ukrainian news website. In it, Zelenskiy told Ukrainian troopers to spread down their weapons and capitulate to Russian armies. But the video turned out to be a gift of deepfake technology, a segment of artificial media designed by machine learning.
Some scientists are currently concerned that identical technology could be utilised to perpetrate research forgery by constructing contrived images of spectra or biological specimens.
‘I’ve been bothered very much about these types of technologies,’ says microbiologist and science probity professional Elisabeth Bik. ‘I believe this is already occurring– constructing deepfake images and publishing [them].’ She questions whether the images in the over 600 completely fabricated studies that she aided expose, which likely came from the same paper mill, may have been AI-generated.
Unlike manually influenced images, AI-generated ones could be virtually impossible to notice by eye. In a non-peer-reviewed issue study, a group led by computer scientist Rongshan Yu from Xiamen University in China developed a series of deepfake western blot and cancer images. Two out of three biomedical specialists were incapable to differentiate them from the actual thing.
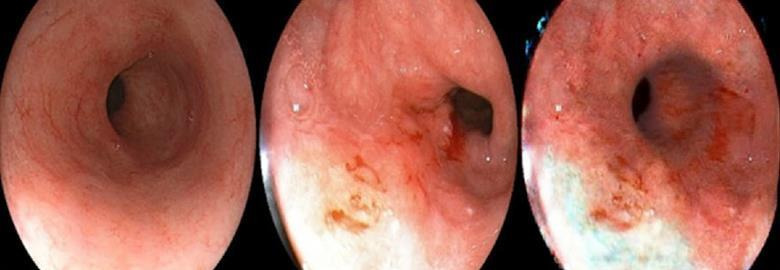
These oesophagal cancer images are deepfakes that were created by a generative adversarial network.
The hardship is that deepfake images are unique, says Yu. They display none of the hints somebody usually look for – reprised elements and environment inconsistencies, for instance. Moreover, ‘deepfake and additional tools are now admiringly unrestricted’, says Yu. ‘It isn’t rocket science, you don’t need the greatest professional in AI to operate them.’
Deepfakes are often established on generative adversarial networks (Gan), where a generator and a discriminator attempt to outcompete each other. ‘One network attempts to develop a fake image from white noise, let’s consider a face,’ illustrates deepfake technology investigator John (Saniat) Sohrawardi from the Rochester Institute of Technology, US. ‘It doesn’t comprehend how to render a face originally, so it bears the support of a discriminator, which is another grid that memories how to tell apart whether an image is genuine or imitation.’ Eventually, the generator will trick the discriminator into believing its images are genuine.
Provided that Gans can forge faces that are indiscernible from genuine ones, ‘I don’t think it should reach as a surprise that it can induce these sorts of fairly prosaic biological images’, says Hany Farid, who specialises in digital forensics and misinformation at the University of California, Berkeley, in the US. But while deepfakes are a menace to be handled thoughtfully, ‘I’m far more concerned about reproducibility, p-hacking, Photoshop manipulation – the old school stuff, which is still going to, I suspect, overpower for quite a while.’
Matthew Wright, director of Rochester’s Global Cybersecurity Institute, conforms. ‘I just don’t find this to be notably fearful, even though it’s technically entirely feasible and presumably challenging to catch if somebody did it.’
The digital artefacts dumped behind by machine learning could be utilised to pinpoint simulated images, exemplifies Farid, though fraudsters usually discover a way around such techniques after merely a few months. ‘In the fate, the superior simple key is the busy ones, authenticate with hard encryption at the end of the recording,’ Farid articulates. He considers that science’s self-correcting tools will ultimately trash bogus analysis.
Yu says it’s murky whether the publications already contain AI-generated pictures. ‘I think we have achieved the juncture where we can no longer denote if the article is genuine or bogus,’ says Bik. ‘We need to operate harder with organisations to have them … take part of the accountability,’ she indicates, and take away stress from investigators whose whole profession might hinge on issuing in an international journal.
Reference
L Wang et al, Patterns, 2022, 3, 100509 (DOI: 10.1016/j.patter.2022.100509)